MathType - The #Gradient descent is an iterative optimization #algorithm for finding local minimums of multivariate functions. At each step, the algorithm moves in the inverse direction of the gradient, consequently reducing
Por um escritor misterioso
Descrição

GRADIENT DESCENT Gradient descent is an iterative optimization algorithm used to find local minima…, by Kucharlapatiaparna
Solved 4. Gradient descent is a first-order iterative

nonlinear optimization - Do we need steepest descent methods, when minimizing quadratic functions? - Mathematics Stack Exchange

Optimization Techniques used in Classical Machine Learning ft: Gradient Descent, by Manoj Hegde
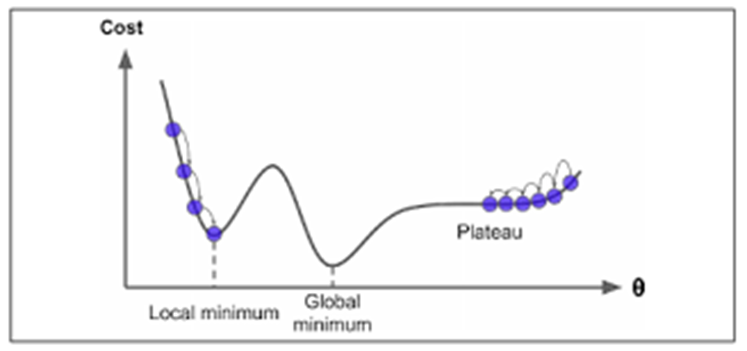
Optimization Techniques used in Classical Machine Learning ft: Gradient Descent, by Manoj Hegde
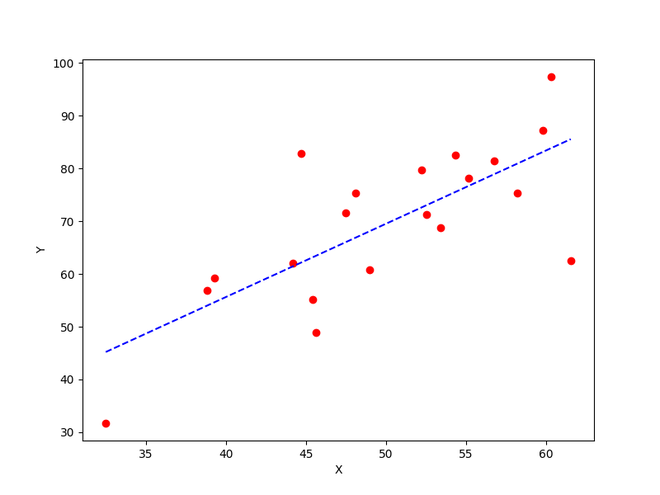
How to implement a gradient descent in Python to find a local minimum ? - GeeksforGeeks

Gradient Descent Algorithm-Chain Rule-Directional Derivative, by Kamil Budagov
Solved] . 4. Gradient descent is a first—order iterative optimisation
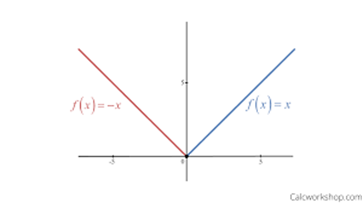
Optimization Techniques used in Classical Machine Learning ft: Gradient Descent, by Manoj Hegde
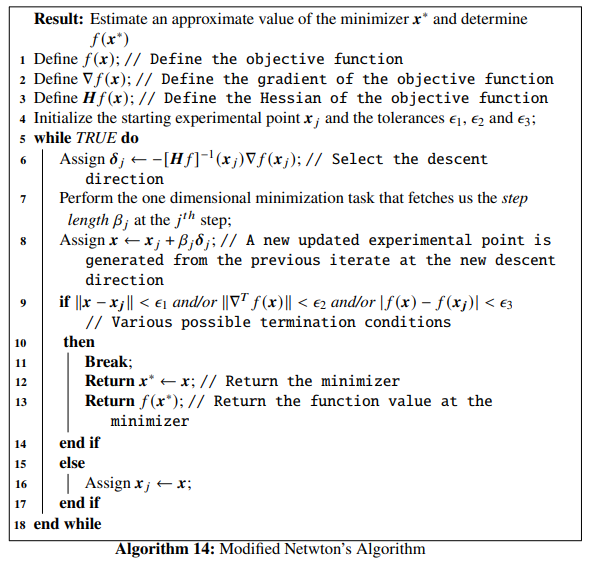
Chapter 4 Line Search Descent Methods Introduction to Mathematical Optimization
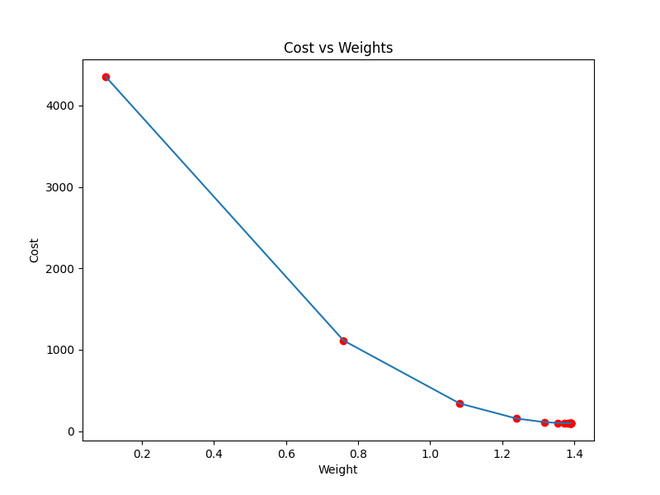
How to implement a gradient descent in Python to find a local minimum ? - GeeksforGeeks
Solved] . 4. Gradient descent is a first—order iterative optimisation
de
por adulto (o preço varia de acordo com o tamanho do grupo)