Applied Sciences, Free Full-Text
Por um escritor misterioso
Descrição
This article focuses on the recent advances in the field of reinforcement learning (RL) as well as the present state–of–the–art applications in games. First, we give a general panorama of RL while at the same time we underline the way that it has progressed to the current degree of application. Moreover, we conduct a keyword analysis of the literature on deep learning (DL) and reinforcement learning in order to analyze to what extent the scientific study is based on games such as ATARI, Chess, and Go. Finally, we explored a range of public data to create a unified framework and trends for the present and future of this sector (RL in games). Our work led us to conclude that deep RL accounted for roughly 25.1% of the DL literature, and a sizable amount of this literature focuses on RL applications in the game domain, indicating the road for newer and more sophisticated algorithms capable of outperforming human performance.
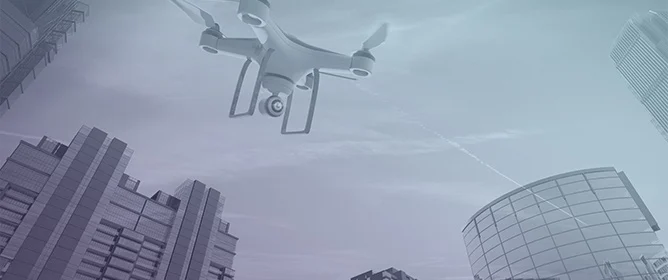
Applied Sciences An Open Access Journal from MDPI
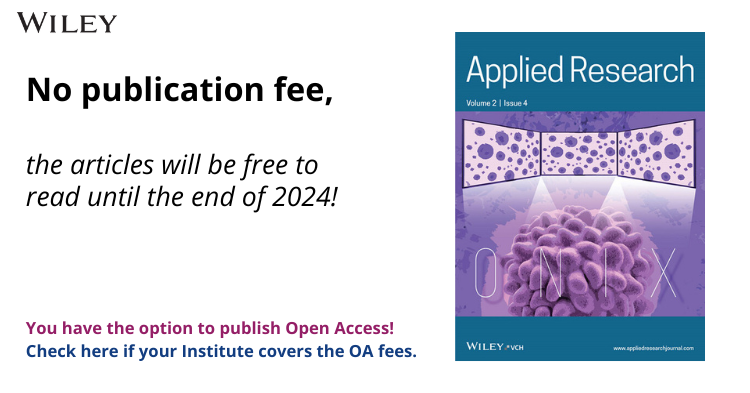
Applied Research - Wiley Online Library
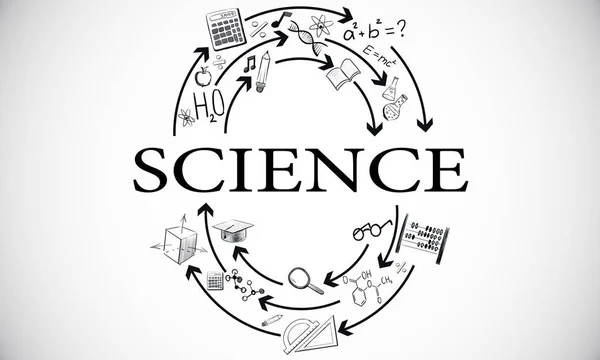
Applied sciences Stock Photos, Royalty Free Applied sciences
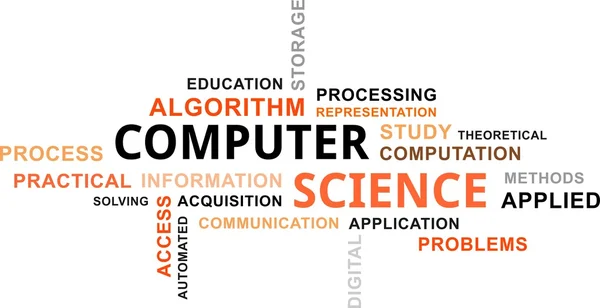
Applied sciences Stock Photos, Royalty Free Applied sciences
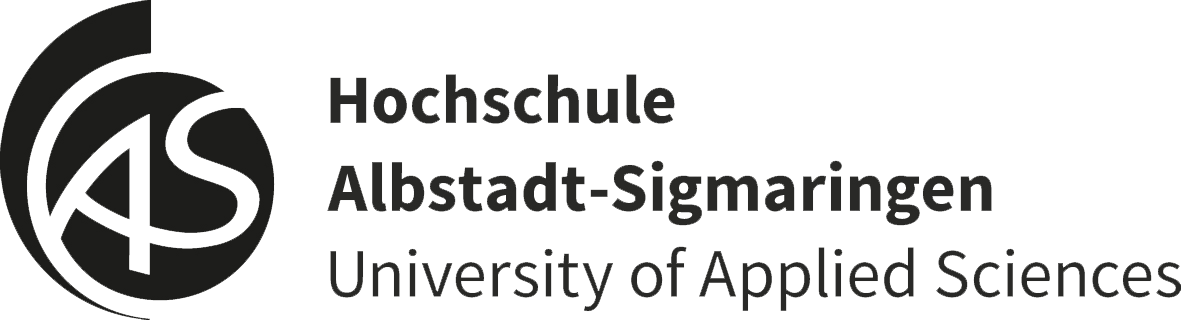
The University of Applied Sciences at Albstadt-Sigmaringen
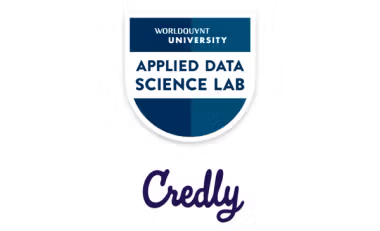
Applied Data Science Lab
Science, health and medical journals, full
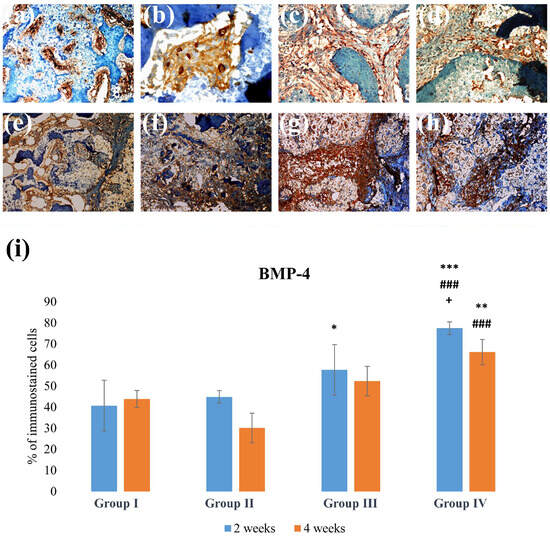
Applied Sciences An Open Access Journal from MDPI
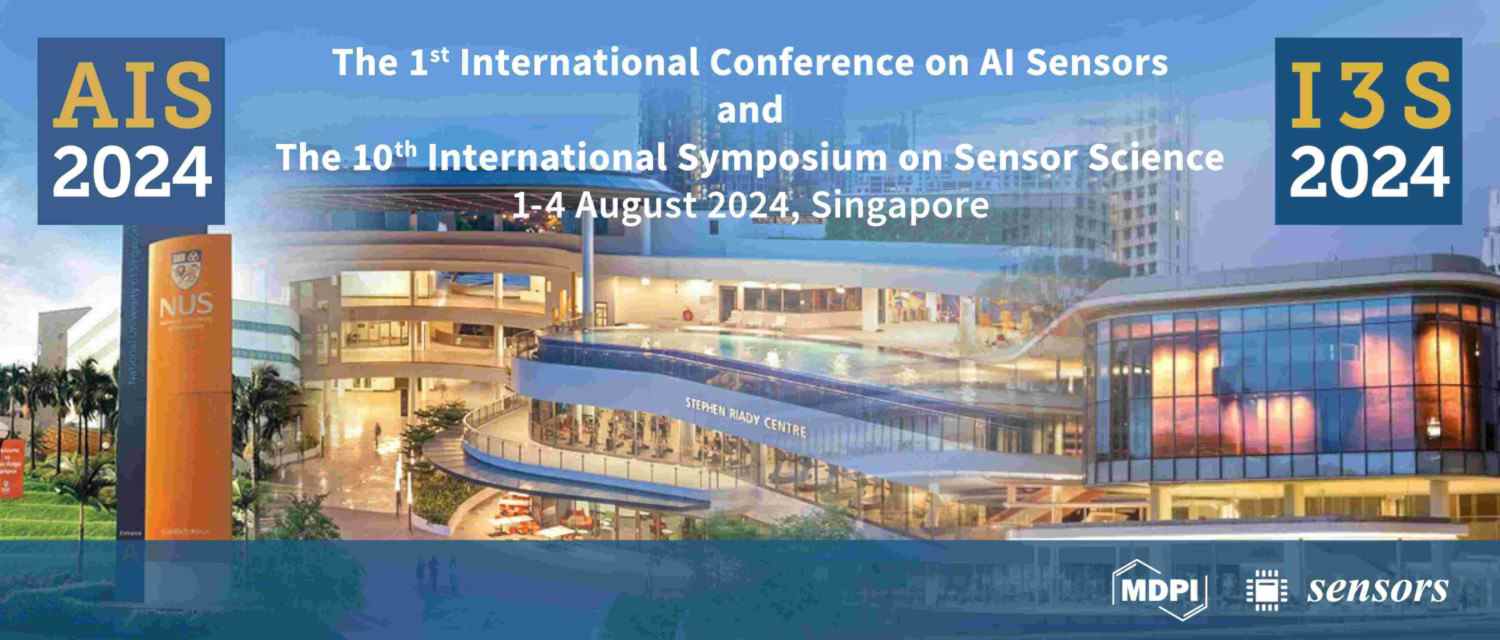
Applied Sciences An Open Access Journal from MDPI

Applied Energy, Journal
de
por adulto (o preço varia de acordo com o tamanho do grupo)